Utilizing machine learning to find reliable and low-cost solar cells
- Researchers at the University of California, Davis College of Engineering are making use of machine learning to identify new materials for high-efficiency solar cells. Making use of high-throughput experiments and also machine learning-based formulas, they have actually found it is possible to anticipate the products' dynamic behavior with really high accuracy, without the demand to carry out as many experiments.
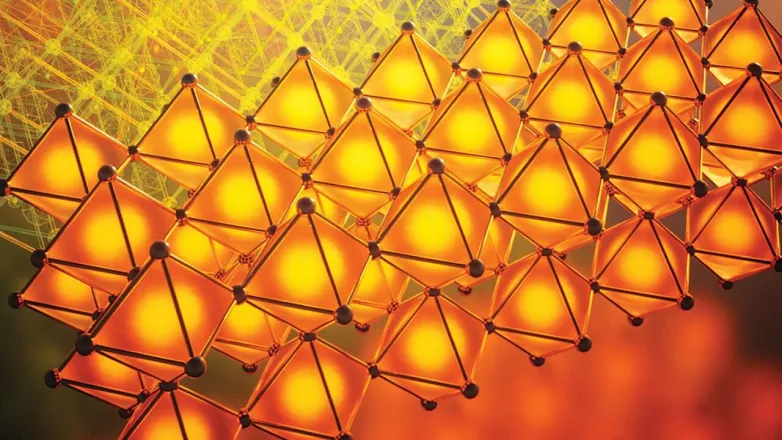
The work is included on the cover of the April issue of ACS Energy Letters.
Crossbreed perovskites are organic-inorganic molecules that have actually obtained a great deal of attention over the past ten years for their prospective usage in renewable resource, said Marina Leite, associate professor of materials scientific research and also engineering at UC Davis and also senior author on the paper. Some are equivalent in performance to silicon for making solar cells, yet they are less costly to make and lighter, potentially allowing a large range of applications, consisting of light-emitting tools.
A primary obstacle in the field is that the perovskite devices often tend to degrade way quicker than silicon when revealed to moisture, oxygen, light, warmth, and voltage. The issue is to find which perovskites incorporate high-efficiency performance with resilience to environmental conditions.
Perovskites have a general structure of ABX3, where A is an organic (carbon-based) or inorganic group, B is lead or tin, and also X is a halide (based on chlorine, iodine or fluorine or a combination). Therefore, "the number of possible chemical combinations alone is enormous", Leite stated. Even more, they require to be examined versus numerous environmental problems, alone as well as in combination, which causes a hyperparameter space that can not be explored using standard trial-and-error methods.
" The chemical criterion space is enormous," Leite stated. "To check them all would be extremely time consuming and also tedious."
High throughput experiments and machine learning
As an initial and also crucial step towards resolving thesechallenges, Leite and also college students Meghna Srivastava and Abigail Hering choose to examine whether artificial intelligence formulas could be reliable when screening as well as predicting the effects of moisture on material destruction.
Srivastava as well as Hering built an automated, high-throughput system to gauge the photoluminescence efficiency of 5 different perovskite films versus the conditions of summer days in Sacramento. They were able to collect over 7,000 measurements in a week, accumulating enough information for a reliable training collection.
They utilized this data to educate three various machine learning algorithms: a straight regression design, a neural network and also an analytical model called SARIMAX. They compared the predictions of the designs to physical outcomes gauged in the laboratory. The SARIMAX model showed ideal efficiency with a 90 percent match to observed results during a home window of 50-plus hours.
"These results show that we can take advantage of machine learning in recognizing candidate products as well as appropriate problems to prevent degradation in perovskites," Leite said. Following steps will be to broaden the experiments to quantify combinations of several environmental aspects.
The perovskite film itself is only a part of a total photovoltaic cell, Leite said. The very same machine learning technique might additionally be utilized to anticipate the habits of a full tool.
"Our standard is distinct, as well as I aspire to see the upcoming measurements. Moreover, I am very happy with the pupils' persistance throughout the pandemic" Leite claimed.
Additional authors on the paper are Yu An and also Juan-Pablo Correa-Baena, both from Georgia Tech.
Also read
- IIT Bombay Pushes 4T Perovskite-Silicon Tandem Efficiency Past 29 Percent
- GCL Sets 29.51 % Record for Large Perovskite-Silicon Tandem Module Efficiency
- Maxwell Raises $273 Million for China Perovskite Tandem Equipment Build-out
- PolyU Tandem Breakthrough Pushes Perovskite-Silicon Solar Cells Beyond Limits Efficiency
- PNE Unveils Wattmate: Revolutionizing Renewable Energy Sales
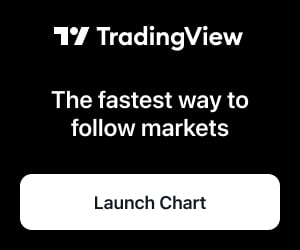