Predicting the device performance of perovskite solar cells with machine learning
- Steel halide perovskite solar cells (PSCs) have been rapidly established in the past years. To obtain high-performance PSCs, it is essential to optimize the construction procedures as well as the structure of the perovskite films. Substantial work has actually been carried out to identify the impacts of the fabrication procedures and also composition of the perovskite films on the device performance.
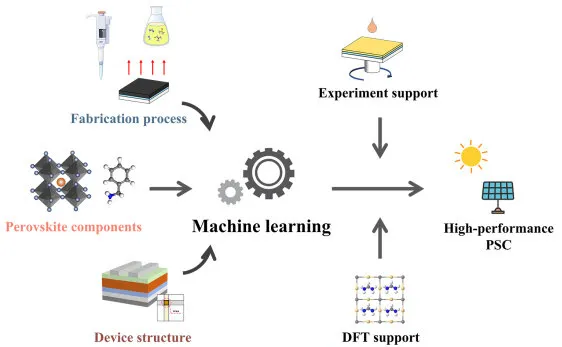
However, it has actually been challenging to elucidate their relationships because of the huge variable room constructed by these factors. Exploring these connections is undoubtedly essential to predict the device performance for reliable device optimization. However, owing to the complexity of these elements, it has actually been impossible thus far to carry out this work solely with trial and error.
Lately, Professor Zheng Xu and also Associate Professor Dandan Song of Beijing Jiaotong University adopted the machine learning (ML) strategy to discover these connections by finding out the existing experimental results, thus making it possible for the prediction of the device performance from these elements.
The effects of these factors on the device performance were evaluated by shapley additive explanations (SHAP) analysis. Moreover, to improve the interpretability of the ML design, the writers thought about A-site cations as an instance to describe as well as verify the predicted results by thickness useful theory (DFT) computations and experiments. This work thoroughly elucidates exactly how ML guides device optimization, consequently also serving as a guide for the reverse style of experiments to obtain high-performance PSCs.
This research study was released in the Journal of Energy Chemistry.
Also read
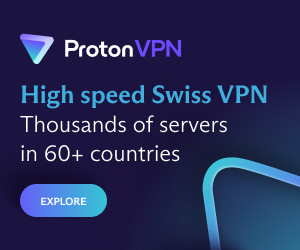