Engineers enlist AI to aid scale up innovative solar cell production
- Perovskites are a household of materials that are currently the leading challenger to potentially replace today's silicon-based solar photovoltaics.
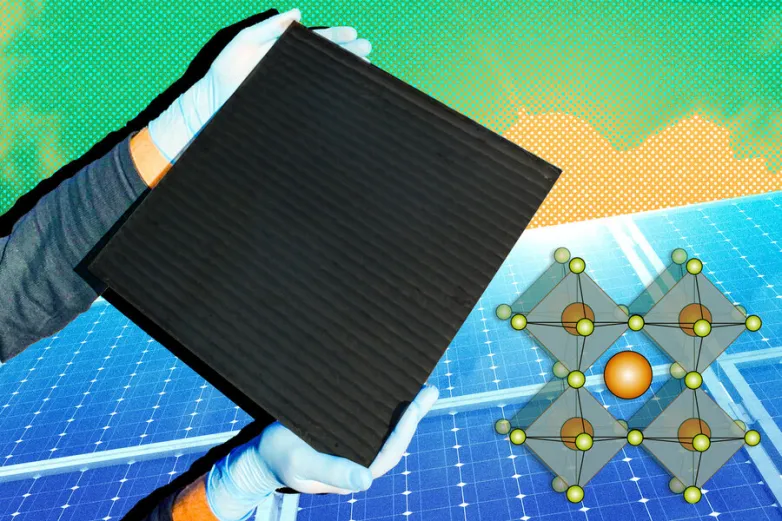
They hold the guarantee of panels that are far thinner and also lighter, that could be made with ultra-high throughput at room temperature level rather than at numerous degrees, and that are less costly and much easier to carry and also mount. Yet bringing these materials from controlled laboratory experiments into a product that can be produced competitively has been a long struggle.
Manufacturing perovskite-based solar cells entails optimizing at the very least a lots or two variables at the same time, also within one certain manufacturing technique among several possibilities. Yet a brand-new system based on an unique strategy to machine learning could quicken the development of optimized manufacturing methods and aid make the future generation of solar power a truth.
The system, developed by researchers at MIT and also Stanford University over the last couple of years, makes it possible to integrate data from prior experiments, and also information based on personal monitorings by skilled workers, right into the machine discovering process. This makes the outcomes more precise and also has actually currently caused the manufacturing of perovskite cells with an energy conversion effectiveness of 18.5 percent, a competitive level for today's market.
The research study is reported today in the journal Joule, in a paper by MIT professor of mechanical design Tonio Buonassisi, Stanford professor of materials scientific research and engineering Reinhold Dauskardt, recent MIT research study assistant Zhe Liu, Stanford doctoral grad Nicholas Rolston, as well as 3 others.
Perovskites are a team of split crystalline substances defined by the arrangement of the atoms in their crystal lattice. There are thousands of such possible compounds as well as several ways of making them. While many lab-scale advancement of perovskite materials makes use of a spin-coating technique, that's not practical for larger-scale manufacturing, so business and also labs worldwide have actually been looking for means of equating these lab products into an useful, manufacturable item.
" There's always a huge obstacle when you're attempting to take a lab-scale process and afterwards transfer it to something like a startup or a production line," says Rolston, who is now an assistant professor at Arizona State University. The group checked out a process that they really felt had the greatest potential, a method called rapid spray plasma handling, or RSPP.
The manufacturing process would certainly include a relocating roll-to-roll surface, or series of sheets, on which the precursor options for the perovskite compound would certainly be splashed or ink-jetted as the sheet rolled by. The material would certainly then move on to a treating phase, offering a rapid and also continuous outcome "with throughputs that are higher than for any other solar modern technology," Rolston says.
" The genuine innovation with this platform is that it would allow us to scale in a manner that nothing else product has allowed us to do," he adds. "Also materials like silicon need a lot longer duration as a result of the handling that's done. Whereas you can think about [this method as even more] like spray painting."
Within that process, at least a lots variables may impact the end result, some of them extra controlled than others. These consist of the structure of the beginning products, the temperature, the humidity, the speed of the handling path, the distance of the nozzle utilized to spray the material onto a substrate, as well as the methods of healing the product. A lot of these factors can interact with each other, and if the process remains in outdoors, then humidity, for instance, may be uncontrolled. Examining all possible mixes of these variables through experimentation is impossible, so machine learning was required to assist guide the experimental process.
But while a lot of machine-learning systems use raw data such as measurements of the electrical as well as various other residential properties of test samples, they do not typically integrate human experience such as qualitative observations made by the experimenters of the visual and also other residential properties of the examination samples, or information from other experiments reported by various other researchers. So, the group located a means to include such outside information right into the machine discovering model, utilizing a likelihood factor based upon a mathematical technique called Bayesian Optimization.
Using the system, he says, "having a model that comes from speculative data, we can discover fads that we weren't able to see before." As an example, they at first had trouble changing for unchecked variations in humidity in their ambient setup. Yet the design revealed them "that we might conquer our humidity obstacles by altering the temperature, as an example, as well as by altering a few of the other handles."
The system currently allows experimenters to a lot more rapidly guide their process in order to optimize it for a provided collection of conditions or required outcomes. In their experiments, the group concentrated on optimizing the power result, but the system can also be utilized to all at once incorporate various other criteria, such as price and durability-- something members of the group are remaining to work on, Buonassisi says.
The scientists were motivated by the Department of Energy, which funded the work, to commercialize the technology, as well as they're currently focusing on technology transfer to existing perovskite suppliers. "We are reaching out to companies now," Buonassisi says, and the code they established has been made openly offered with an open-source web server. "It's currently on GitHub, any person can download it, anybody can run it," he says. "We're happy to help firms get started in using our code."
Already, several business are preparing to create perovskite-based solar panels, although they are still exercising the information of how to produce them, says Liu, who is now at the Northwestern Polytechnical University in Xi'an, China. He says business there are not yet doing large-scale manufacturing, yet rather starting with smaller sized, high-value applications such as building-integrated solar tiles where look is necessary. Three of these companies "get on track or are being pushed by capitalists to produce 1-meter by 2-meter rectangle-shaped modules [equivalent to today's most usual photovoltaic panels], within two years," he says.
' The issue is, they do not have a consensus on what making modern technology to make use of," Liu says. The RSPP method, established at Stanford, "still has a good chance" to be affordable, he says. And also the artificial intelligence system the team developed could show to be important in guiding the optimization of whatever process winds up being used.
" The primary goal was to accelerate the process, so it required less time, less experiments, and much less human hours to establish something that is usable right away, totally free, for industry," he says.
" Existing deal with machine-learning-driven perovskite PV fabrication largely focuses on spin-coating, a lab-scale technique," says Ted Sargent, University Professor at the University of Toronto, who was not related to this work, which he says shows "an operations that is easily adapted to the deposition techniques that dominate the thin-film industry. Just a handful of groups have the simultaneous knowledge in engineering as well as computation to drive such breakthroughs." Sargent adds that this approach "could be an exciting development for the manufacture of a wider family of products" including LEDs, various other PV technologies, as well as graphene, "basically, any kind of sector that makes use of some form of vapor or vacuum deposition."
Also read
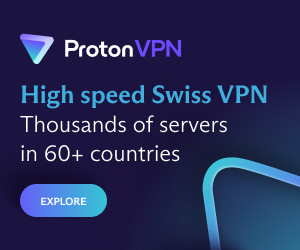