Revolutionizing Solar Cell Development with Machine Learning
- Unlocking the potential of perovskite solar cells with machine learning and data mining techniques for optimized efficiency and renewable energy advancement.
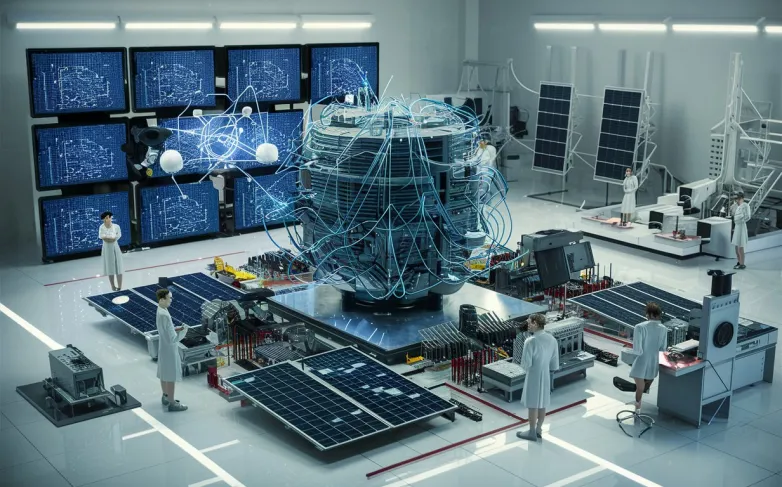
Researchers from Shanghai Jiao Tong University, Shanghai University of Electric Power, and Shandong Normal University are using machine learning and data mining techniques to streamline the development of high-efficiency perovskite solar cells (PSCs). By predicting key features like perovskite bandgap and electron transport layer thickness, the team aims to optimize preparation processes and advance renewable energy technology. They utilized a dataset of 43,612 PSC compositions from The Perovskite Database Project and employed an interpretable ML approach to analyze the impact of various parameters on high PCE prediction.
The research provides insights into the bandgap prediction of perovskite materials and the influence of different descriptors on high-efficiency PSCs. By combining cutting-edge ML techniques with a goal-driven framework, the team aims to accelerate the development of efficient PSCs and shed light on the optimization parameters affecting their performance. This approach offers a systematic way to understand the complex relationships between features and PCE, ultimately contributing to the renewable energy sector's growth.
How are researchers using machine learning to enhance perovskite solar cell development?
- Researchers are using machine learning and data mining techniques to streamline the development of high-efficiency perovskite solar cells (PSCs).
- They are predicting key features such as perovskite bandgap and electron transport layer thickness to optimize preparation processes and advance renewable energy technology.
- The team utilized a dataset of 43,612 PSC compositions from The Perovskite Database Project to analyze the impact of various parameters on high PCE prediction.
- The research provides insights into bandgap prediction of perovskite materials and the influence of different descriptors on high-efficiency PSCs.
- By combining cutting-edge ML techniques with a goal-driven framework, the team aims to accelerate the development of efficient PSCs and shed light on optimization parameters affecting their performance.
- This approach offers a systematic way to understand the complex relationships between features and PCE, ultimately contributing to the growth of the renewable energy sector.
Also read
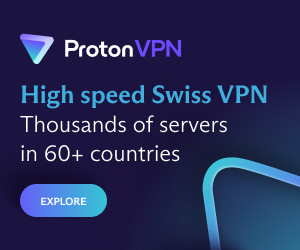