New methods for fault detection revealed at EU PVSEC
Sep 11, 2019 09:00 PM ET
- Quality control and problem detection and classification was brought into focus at the conference in Marseille. A packed house at a session focused on the latest fault detection techniques indicated the high level of interest in the field.
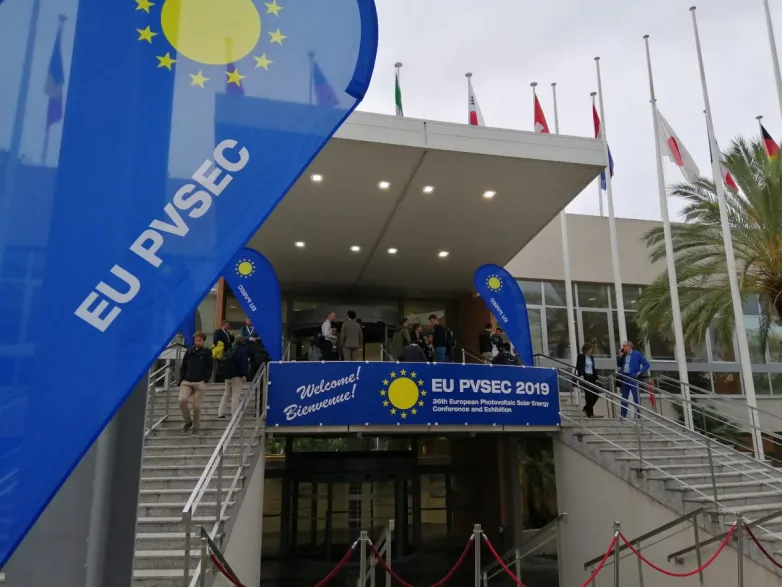
Several new approaches are emerging in the drone and thermal imaging techniques used to detect module and string level faults in utility scale PV arrays. The application of artificial intelligence (AI) and machine learning to the field is also developing as the volume of data informing models rises and becomes more accurate.
Jay Lin, from Taiwan-based PV Guider presented examples of ultraviolet fluorescence (UVFL) inspections. The method uses drones to illuminate PV modules with UV light at night.
Lin explained how the technique can identify cell problems. “All the cracks in the EL [electroluminescence] imaging can be seen on the UVFL,” he said. “You will see the dark area [on the image captured by the drone] along the cracks.”
At a height of 4.5m, UVFL inspection encompasses 16 modules in each image. Closer in, Lin’s team found too few modules were captured in each image. At 6.5m, cracks only showed up in the middle of the image. At 9m, cracks were not visible as the UV light was too weak.
One of the major advantages of UV illumination is that the age of cell cracks can be determined. The cracks show up on the UV image as a dark area which becomes more pronounced as the defect ages.
Lin also conceded limitations to UVFL, in that fluorescence is not evident in the UV imaging of glass-glass modules or when high transmission EVA is a feature.
Informing maintenance decisions
The use of aerial infrared thermography to screen for and quantify failures at PV power plants was also discussed at the session. Stijn Scheerlinck, from Engie, set out his experience from the perspective of a company constructing and operating 3 GW of large scale solar assets.
Scheerlinck explained how aerial thermography can be an important tool for the allocation of resources on the ground. “As an operator, when you are confronted with a performance issue, then there is a complex set of possible origins that are not contributing individually but sometimes strengthening each other,” he said. Hence his team deployed aerial thermography, along with a failure analysis model, to help inform the decision as to whether to send technicians to the site. “It is a first state-of-health method not only for one asset but for a fleet of assets,” he said.
Engie conducted analysis of a portfolio of 20 MW of utility scale solar generation assets alongside drone operator Sitemark in various European locations. The energy company concluded such an approach was helpful in making an “early qualification” of potential problems and could be effective in informing decisions to take potentially costly on-the-ground interventions.
The application of artificial intelligence and self-learning algorithms was also highlighted at the EU PVSEC session. Chantelle Clohessy, from Nelson Mandela University in Port Elizabeth, South Africa; and A.K Vidal de Oliveira, from Brazil’s Fotovoltaica UFSC solar institute, presented their work on deploying machine learning for fault detection in thermal imaging.
The result, as more data is fed into the AI models, is that the accuracy of machine identification and classification of various faults increases by up to 90%. The replacement of human processing of images with machines has the potential to vastly decrease processing times, a particularly useful feature when assessing megawatt-scale arrays.
Also read
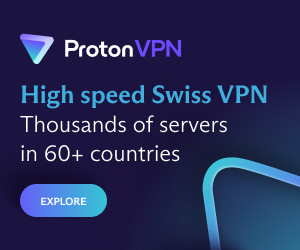