New algorithm to limit the huge list of prospective batt materials
- A group of American scientists have selected 133 thousand prospective molecules for batt electrolyte from the list of 166bn with the help of newly created machine learning algorithm. The new approach is said to minimize the cost of computational examination without compromising the investigation accuracy.
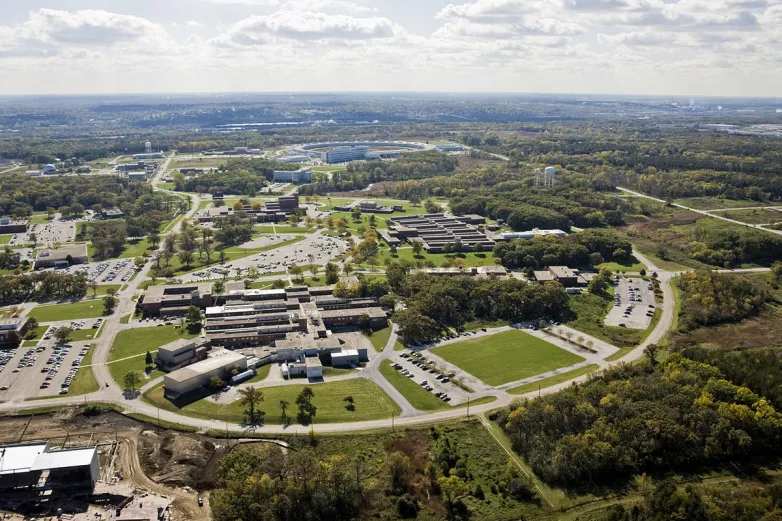
Scientists from all over the globe have long been looking for alternative materials to substitute ion and lithium commonly used in batteries. The new batt has to be at least as efficient as Li-ion and at the same time be deprived of such drawbacks as use of costly expensive materials, gradual efficiency decrease and explosion hazard.
So far, a few technologies have proven promising and are likely to be introduced into commercial use. However, lots of prospective materials have not been thoroughly examined yet. So there are still a lot of opportunities for a new high-potential discovery.
Unfortunately, the vast diversity of unstudied materials means the research is likely to take enormous time and effort, taking into account the limited technical capabilities and high costs of investigation.
Keeping the above in mind, a research team from U.S.-based Argonne National Lab decided to narrow down the list of 166bn molecules subject to investigation. They have created and applied an AI-based algorithm to select 133 thousand molecules most likely to suit the purpose.
The narrowed list of 133 thousand molecules has then been processed with the more advanced computational model – G4MP2. The results of the calculation will be used for selecting the molecules worth particularly thorough examination.
Also read
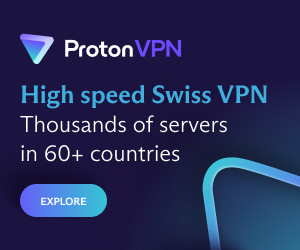