Neural networks improving solar power forecasting
- An international research team has developed a new approach for solar power forecasting that combines neural networks and pattern sequences for the first time. The performance of the new Pattern Sequence Neural Network (PSNN) was tested on an Australian data set that includes information from two years of forecasts. It can be used with different clustering and cluster-sequence extraction algorithms, and can be applied to multiple related time sequences
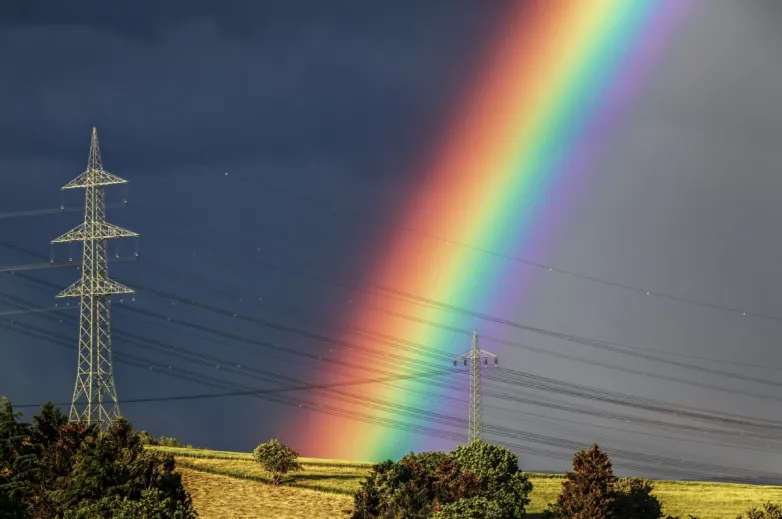
If you have ever asked yourself why Google Translate has become much better at translating in recent years, the answer is because of artificial neural networks.
Since November 2016, the company’s online translation tool has relied on a neural machine translation (NMT) system. It is based on example-based machine translation, which helps the system learn from millions of examples in its database. The system also now allows direct translation from one language to another, while the earlier versions of Google Translate needed to first translate text into English before it could be translated into another language.
All in all, artificial neural networks have provided tangible improvements – a significant technological leap forward. Artificial neural networks are a machine learning technology that work in a similar way to the human brain.
This technology also has many potential applications in the field of artificial intelligence and, because AI is now widely used for energy management and analysis, it’s ideal for the solar energy business, too. Researchers from Australia’s University of Sydney and Spain’s University Pablo de Olavide, for example, have proposed a new approach for solar power forecasting that combines neural networks and pattern sequences for the first time.
The novelty of this new study is adding the powerful effects of artificial neural networks to that of pattern sequences. In mathematics, pattern sequences are commonly used to predict what comes before or after a set a numbers arranged in a particular order, and they have already been used in forecasting for PV power generation. The approach, described in the paper Pattern Sequence Neural Network for Solar Power Forecasting, is based on the idea that it is possible to build a separate prediction model for each pattern sequence type.
The Pattern Sequence Neural Network (PSNN) proposed by the scientists was compared to Pattern Sequence-based Forecasting (PSF), which is a forecasting method combining clustering and sequence matching than is also based on pattern sequencing, but does not work with neural networks. According to the scientists, the PSNN combines the efficient pattern-sequence extraction and similarity-matching of the PSF algorithms with the advantages of neural networks to model complex and nonlinear relationships.
“PSNN is a general approach that can be used with different clustering and cluster sequence extraction algorithms, and can be applied to multiple related time sequences,” the researchers explained.
The performance of the approach was tested on an Australian data set comprising information from PV solar, weather and weather forecast over a period of two years. The PV data was collected from a rooftop array at the University of Queensland in Brisbane.
“The raw PV data was measured every 1 minute and was aggregated to 30-minute intervals by taking the average value of the interval,” the research group said.
The PSNN, which can be applied to multiple complementary time series, works by taking a sequence of cluster labels and extracting different pattern sequences to build a separate NN prediction model for each of them. The tests have shown that all PSNN models outperform their corresponding PSF models in solar power forecasting.
“All PSNN versions outperformed the PSF methods, and the differences were statistically significant,” the researchers said.
The research team now plans to determine whether seasonal prediction models can improve the accuracy of this approach, or whether these methods can be applied to other forecasting tasks.
Also read
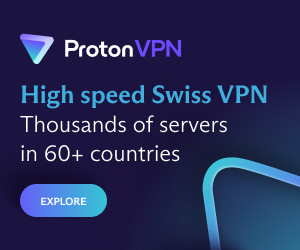