AI-driven robots are making new materials, improving solar cells and other technologies
- Ada, an AI-driven robot, searches for new solar cell designs at the University of British Columbia.
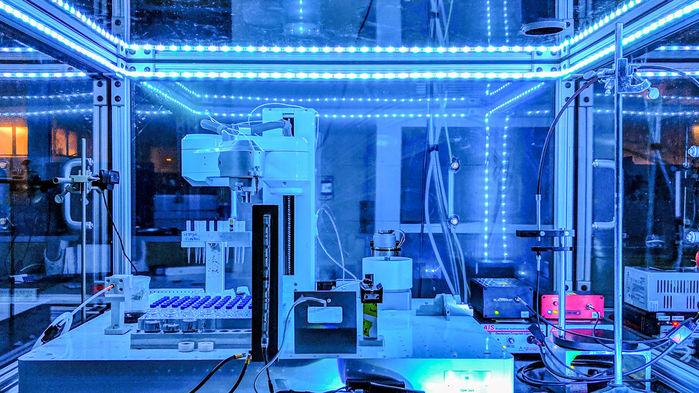
In July 2018, Curtis Berlinguette, a materials scientist at the University of British Columbia in Vancouver, Canada, realized he was wasting his graduate student's time and talent. He had asked her to refine a key material in solar cells to boost its electrical conductivity. But the number of potential tweaks was overwhelming, from spiking the recipe with traces of metals and other additives to varying the heating and drying times. "There are so many things you can go change, you can quickly go through 10 million [designs] you can test," Berlinguette says.
So he and colleagues outsourced the effort to a single-armed robot overseen by an artificial intelligence (AI) algorithm. Dubbed Ada, the robot mixed different solutions, cast them in films, performed heat treatments and other processing steps, tested the films' conductivity, evaluated their microstructure, and logged the results. The AI interpreted each experiment and determined what to synthesize next. At a meeting of the Materials Research Society (MRS) here last week, Berlinguette reported that the system quickly homed in on a recipe and heating conditions that created defect-free films ideal for solar cells. "What used to take us 9 months now takes us 5 days," Berlinguette says.
Other material scientists also reported successes with such "closed loop" systems that combine the latest advances in automation with AI that directs how the experiments should proceed on the fly. Drug developers, geneticists, and investigators in other fields had already melded AIs and robots to design and do experiments, but materials scientists had lagged behind. DNA synthesizers can be programmed to assemble any combination of DNA letters, but there's no single way to synthesize, process, or characterize materials, making it exponentially more complicated to develop an automated system that can be guided by an AI. Materials scientists are finally bringing such systems online. "It's a superexciting area," says Benji Maruyama, a materials scientist with the U.S. Air Force Research Laboratory east of Dayton, Ohio. "The closed loop is what is really going to make progress in materials research go orders of magnitude faster."
With more than 100 elements in the periodic table and the ability to combine them in virtually limitless ways, the number of possible materials is daunting. "The good news is there are millions to billions of undiscovered materials out there," says Apurva Mehta, a materials physicist at the Stanford Synchrotron Radiation Lightsource in Menlo Park, California. The bad news, he says, is that most are unremarkable, making the challenge of finding gems a needle-in-the-haystack problem.
Robots have already helped. They are now commonly used to mix dozens of slightly different recipes for a material, deposit them on single wafers or other platforms, and then process and test them simultaneously. But simply plodding through recipe after recipe is a slow route to a breakthrough, Maruyama says. "High throughput is a way to do lots of experiments, but not a lot of innovation."
To speed the process, many teams have added in computer modeling to predict the formula of likely gems. "We're seeing an avalanche of exciting materials coming from prediction," says Kristin Persson of Lawrence Berkeley National Laboratory (LBNL) in California, who runs a large-scale prediction enterprise known as the Materials Project. But those systems still typically rely on graduate students or experienced scientists to evaluate the results of experiments and determine how to proceed. Yet, "People still need to do things like sleep and eat," says Keith Brown, a mechanical engineer at Boston University (BU).
So, like Berlinguette, Brown and his colleagues built an AI-driven robotics system. Their goal was to find the toughest possible 3D-printed structures. Toughness comes from a blend of high strength and ductility, and it varies depending on the details of a structure, even if the material itself doesn't change. Predicting which shape will be toughest isn't feasible, Brown says. "You have to do the experiment."
As a test case, the BU team set out to make salt shaker–size, barrel-shaped structures from a plastic. They varied the number of struts that make up the outer wall of the barrel and details of each strut's shape and orientation. Testing all possible combinations, about a half-million, wasn't realistic. So, they initially had their robots fabricate 600 structures that sampled the full array of options. A kind of vise then squeezed each one until it gave way.
The group then added an AI decision-making algorithm that calculated the most likely next best design after each test. The program spots trends in attributes that confer toughness, such as the thickness and radius of each strut, in order to predict even sturdier structures. "We basically turned on the machine and walked out the door," Brown says. After 24 hours and just over 60 designs, the AI-driven system had come up with a tougher barrel than any of the original designs.
Many more closed loop efforts were showcased at the MRS meeting. Researchers at the Massachusetts Institute of Technology in Cambridge and LBNL have independently developed autonomous systems to find better perovskite photovoltaics—cheap, lightweight materials that are poised to revolutionize solar energy. A team at Carnegie Mellon University in Pittsburgh, Pennsylvania, reported using another AI system to find safer charge-carrying electrolytes for lithium-ion batteries, which are now prone to catching fire. And researchers at the University of Liverpool in the United Kingdom have developed a suite of AI-driven robots to discover novel catalysts for generating hydrogen gas, a potential carbon-free fuel, from water.
Few of these projects have turned up blockbuster results, researchers acknowledge. However, Maruyama says, "It's still early days." One challenge is that materials scientists themselves often don't agree on how best to relate a material's conductivity or other testable properties to its structure, says John Gregoire, a physicist at the California Institute of Technology in Pasadena. "If we haven't figured out how to break that down in the community, it's hard to imagine how we will teach a computer to do it," he says.
Another issue is that each team must design its own robotics and software systems, as standards have yet to take shape. "Everyone is exploring different ways to do this," says Joshua Schrier, a computational chemist at Fordham University in New York City. Eventually, the materials community may coalesce around a handful of systems that can be used by a wide swath of researchers, Schrier says. "Over the next year or two I think we'll begin to see things converge."